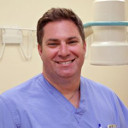
- Application developers should realize AI is currently functioning like a high school sophomore in a gifted class and plan accordingly
- AI will begin its role in Medicine as a tool to make human doctors better
- Safe and effective use of AI requires supervision
- Doctors will require extensive training to safely and effectively onboard AI
“Well, all of you are going to be allopathic medical doctors. What’s that good for?” The challenge was laid down by Dr. Andrew Weil, lecturing to my second-year Medical School class at the University of Arizona in 1989. My first thought was, “Cool, this guy looks like Modern Moses, and being a doctor makes you good at pretty much everything.” Over the next hour Andy systematically disabused me of the second part of notion. He laid out the outcome of various medical conditions over the century in which Allopathic medicine came to preeminence. Lifesaving progress in trauma, pharmaceutical management of chronic and infectious disease, not as much as hoped in many of the worst cancers. It was a great lecture, because it showed the value of jumping out to 30,000 feet to become aware of our capabilities, and limitations.
We have a long history in Medicine of placing hope cart far in front of reality horse. When I was an undergraduate student at Pepperdine I was told not to consider surgery because advances in drug testing paradigms and recombinant DNA produced pharmaceuticals were going to make surgery obsolete. They didn’t. As an NIH Lab Director in the early 90’s molecular biology showed the potential of gene therapy to alter the core mechanism of disease and seemed destined to reverse not only congenital disease but to cure cancer itself. But 30 years later I’m getting ready to retire from my career in surgery, and I can count on one hand the number of significant diseases effectively treated using those ideas. Before wasting decades, this may be a good time to take the converse of Dr. Weil’s approach to cut through the hype and ask what is AI, and how should it NEVER be used in medicine?
What is AI?
For the purposes of this article AI is the use of computer programs to solve problems and perform tasks that traditionally required human intelligence. Machine learning is a tool of AI in which computers use algorithms to learn and improve from their own experience. Over the last decade a lot of the excitement around AI justifiably comes from its ability to solve some vexing problems that were previously very challenging for computers, such as identifying objects in pictures (computer vision), transcribing and interpreting speech (speech recognition), characterizing handwriting, filtering e-mail spam, playing games like chess, picking the right people to date, estimating real estate values, and driving a car. While these problems were certainly very challenging for computers, they are typically addressed and mastered by humans at a relatively young age. Speech recognition begins in infancy; most humans can talk by age 3. Kids usually learn to write in pre-school and become well accomplished by junior high. They usually learn to drive in High School. So, if you think about it, at this point AI is like a high school sophomore in a gifted program. AI can read, write, talk, watch YouTube; spends a lot of time thinking about dating, is great at memorization and games; but if you drive around Chandler Arizona a lot, like I do, you know we have a way to go before you’d trust AI with your car keys on a rainy Saturday night.
Application Human Age of Acquisition
- Computer Vision 1
- Speech Recognition 2
- Handwriting Characterization 4
- Spam filtering 8
- Chess 10
- Dating 14
- Driving 16
If you were putting together a moon-shot program to cure cancer, would you depend entirely on high school students to fly the rocket? Probably not (parents of adolescents who find shooting your offspring into space in a rocket attractive don’t count). Effective uses of AI in Medicine require us to focus on the real capabilities of AI and how we can best deploy it to help patients. In doing so, here are five rules we should following to be sure we get the best and safest results.
#1. Never leave AI alone with the patient
Machine learning models are great at processing large volumes of data, but they don’t have insight into the limitations of their paradigm. Amazon just announced new software which extract features from hundreds of thousands of medical records in the time I can do one. But I’m really good at knowing when to pump the brakes when things are getting wonky. For example, a bot which determines whether patients with osteoarthritis of the spine are ready for a procedure may be clueless that its looking at a case of patient with a traumatic injury which will get better by itself, superimposed on a long smoldering case of arthritis; or that the pain is actually coming from the hip not the spine, or that the patient is exaggerating his symptoms to gin up the settlement of a lawsuit; or that the patient is really drug addicted and is perpetuating his symptoms to get opiates.
Machine learning purists would point out that exceptions can be dealt with by pre-processing the data. And to a great extent they’re right. But tell that to the lady walking her bike across the road in Tempe when it turned out the Uber Self Driving Vehicles weren’t ready to recognize ladies walking their bikes at night. You can’t tell her, because she’s dead. Even though there was someone watching. Nothing worthwhile is ever easy. There will certainly be hard lessons along the way. But in the meantime, human involvement in the decision-making process seems the best way to protect patients while we iteratively improve the AI. There will be a ramp up period where we essentially need to “onboard” AI to the healthcare delivery team. In the meantime, don’t leave the AI alone with the patients.
#2. Never use a Convolutional Neural Network when a Random Forest will do
Convolutional neural networks are a powerful tool for problem solving. The networks mimic the architecture of the human brain, with “neurons,” that are weighted in a feed forward manner by data which represents features extracted from real life scenarios. By dividing the data in a training set and a testing set we can establish some mathematical measures of the model’s ability to make decisions. We can even establish the old faithful sensitivity and specificity variables familiar to most physicians. But we have no real way to explain how the decision was made in an individual case. That’s a problem.
Patients need to understand their condition and the risks, benefits, and alternatives to be able to make their best decision regarding alternatives for treatment. It’s not going to help Parents to explain that the neural network examined their two-year-old and the local minimum for the loss risk function was found at tonsillectomy. It would help to be able to state the exact risks to the toddler of having the procedure.
Fortunately, there are other AI tools which will likely be easier to explain to patients. Random forest models can be programmed around specific outcome parameters with results that are more approachable. It’s not hard to imaging saying to a patient “Mrs. Jones, we examined 50 thousand cases like yours and determined that the risk of infection, which could be life threatening to you, is 28% Are you sure you want to have this procedure, knowing the risk is that high? We think there are better and safer alternatives for you.”
#3. Never trust a monkey with a scalpel
I did my Neurosurgery training at the University of California San Francisco. My Chairman, Dr. Charlie Wilson, would sometimes drop pearls on us Resident Physicians like “I could teach a monkey to operate better than you, but the monkey keeps contaminating himself by touching his mask.” Today that sounds abusive; in the 90’s he was trying to reassure us not to worry about our ultimate surgical abilities: if a monkey can do it, you can too!
But the point is actually useful today. In the ramp up period AI should assist and advise, not be given a scalpel. AI needs to be embedded into care delivery in ways that optimize its strengths and minimize its weaknesses. A good example was embedding IBM’s Watson into hospital tumor boards. Watson was able to bring instant access to decades of chemotherapy trials to the discussion. And IBM reports that over time, the human doctors and Watson began to converge on their recommendations. Great minds (eventually and with lots of interaction) think alike.
#4. Never let AI break the bad news
As a neurosurgeon I’ve held hundreds of hands of loved ones as they broke down in tears with the realization that the person they loved more than anyone else in the world was dead or surely dying. If we try over time, we will make AI more humane, but not human. In 1950 Alan Turing proposed the ultimate test for AI would be when its outputs were indistinguishable from humans’. In the middle of the night in the Emergency Room after a fatal car accident, that test will never be passed. Some jobs will need to be left to the people.
But AI can help. I can’t calculate in real time the fluctuating probability of survival given fifty changing indicator variables. I’m also pretty hard pressed to determine the optimal weights to assign those variables given a population of patient survival data to process. To make matters worse, I’m likely to become emotionally biased by the patient interaction, and I’m prone to focus on my specialty over the big picture. My limitations and bias may lead to procedures that don’t really stand a chance of being successful. AI can be used to help me overcome these limitations.
#5. Never underestimate the potential of training
You know that moment in “dog training” when you realize who’s really being trained and it’s not the dog? That’s likely to happen with AI, too. As machine learning algorithms proliferate, Doctors are going to need formal training to get the most out of AI in practice. Fortunately, we were able to get some good experience integrating robots into clinical practice. Formal education regarding the capabilities, limitations, and pitfalls of the technology are essential in the onboard.
And that’s great because the dream team in healthcare will be the combination of AI and doctors. AI is good at everything we’re bad at, and bad at the things we do best. They’re the perfect partner.